Let’s Talk about Four of the Six Abstracts that will be Published by the EnsoData team at SLEEP 2021
Dive into the brief overviews of our poster-worthy research
If you’re here to read this piece, and you’re looking for a brief overview of FOUR of our SIX abstracts being published at SLEEP this year, then you’re in the right place. From the novel ideas and discoveries based on the latest advancements in Artificial Intelligence (AI) and Machine Learning (ML) technology, the EnsoData research team and our distinguished academic and industry research collaborators really dug into the data this year. With that said, let’s talk through the first FOUR research abstracts we are publishing at SLEEP in 2021.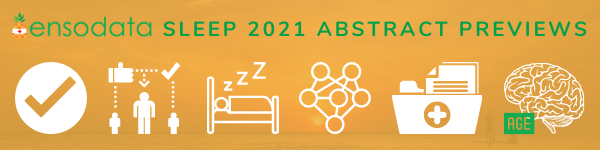
EEG-Based Deep Neural Network Model for Brain Age Prediction
In the study, “EEG-Based Deep Neural Network Model for Brain Age Prediction and its Association with Patient Health Conditions,”our AI team set out to see what we could identify and predict about an individual’s “brain age.” For those unaware of the term, brain age refers to the assessment of the functional and physiological age of one’s brain compared to their real (chronological) age. In patients with certain neurological diseases that affect the central nervous system, the brain’s functionality starts to deteriorate more quickly. In advanced stages of these types of diseases, a person’s brain age might appear to be greater (older) than their real age. Our aim was to find a way to accurately estimate a person’s brain age. Then, by comparing the assessed brain age to the chronological age, a variety of indices can be formulated and potentially used as diagnostic biomarkers of brain health. We were able to train an AI model to predict the age of patients based on the raw EEG signals recorded during a full night polysomnography (PSG) study. And in short, the research was eye-opening. On average, the AI model was able to predict the age of a patient within +/- 4.6 years across 1,172 patients. More than just a close estimation, we were able to observe correlations between varying deviations of brain age from the chronological age and shifts in different diseased and healthy patient populations. For example, diabetic patients expressed a higher mean predicted brain age compared to non-diabetic patients. Similarly, patients with low sleep efficiency revealed a higher mean predicted brain age compared to patients with high sleep efficiency. Overall, dozens of patient disorders, including depression, diabetes, hypertension, severe excessive daytime sleepiness, and low sleep efficiency were correlated with a shift in the predicted brain age from the real age of the patients. The results in this study provide initial evidence for the potential of utilizing AI to assess the brain age of a patient. Our hope is that with continued investigation, research, and clinical studies, a brain age index will one day become a diagnostic biomarker of brain health, much like high blood pressure is a biomarker for risks of stroke and other cardiovascular disorders.PAP Adherence Phenotypes Utilizing Deep Neural Networks and Unsupervised Clustering
For patients that suffer from obstructive sleep apnea (OSA), using a CPAP (continuous positive airway pressure) machine is often one of the best ways to mitigate the detrimental effects. At the same time, PAP adherence is difficult for many patients and in turn, their providers. In order to implement behavioral interventions such as patient outreach and coaching, it is critical to detect early signs and risks of noncompliance to trigger early outreach. With that in mind, our team set out to see if an AI model could forecast when patients are about to fall out of adherence with their PAP device. This led to our “Redefining Positive Airway Pressure (PAP) Adherence Phenotypes Utilizing Deep Neural Networks and Unsupervised Clustering” research study. In short, we wanted our AI to identify as many subgroups of patients, with a goal of finding techniques to improve PAP adherence within the subpopulations. To do this, we analyzed data from over 10,000 patients. We aggregated a year’s worth of PAP usage data, pulling baseline AHI, age, and sex for each patient. We wanted to answer the following: What could this data tell us about PAP usage patterns among different types of patient populations? And then, how can we improve PAP non-adherence forecasting to enable early patient outreach and intervention? While we obviously want to help EVERY patient with their OSA and their CPAP machine usage, efforts should still be focused in the places where the biggest impact can be made. The scatterplot below shows the different groups of compliant v. non-compliant PAP users, with the red dots representing the users most likely to adhere to change. As you can see, there are more than just compliant and non-compliant groups. The results demonstrate that by running usage patterns and additional patient information through an AI model, we are able to identify an additional group of variable users. From there, organizations can better identify the moment where someone moves from the compliant end into the non-compliant end and when to trigger an intervention.Evaluation of AI-Based EMR Screening Tools for Undiagnosed OSA
In the “Evaluation of Electronic Medical Record Artificial Intelligence Screening Tools for Undiagnosed OSA” study, our team looked at the current STOP-bang screening model. While providers continue to rely on the STOP-bang questionnaire as the gold standard for OSA screening, not every patient actually receives a STOP-bang test when they visit the hospital. Sleep navigators improve the odds, but even in the most well-run health systems, some patients will be missed. And we often wonder: is there a way to get more bang for our buck with the current STOP-bang with the power of AI? In other words, can AI expand the ability of the STOP-bang? In our attempt to find an answer, we leveraged a random forest model, utilizing existing data in electronic medical records (EMRs) in tandem with data pulled from past STOP-bang questionnaires. In doing so, we aimed to demonstrate a new screening tool to increase diagnostic sensitivity and specificity and to facilitate discovery of preclinical OSA phenotypes. What we discovered was a potential sensitivity and specificity in determining OSA comparable to standard STOP-bang performance, all from other data points already standardly collected in the EMR. In layman’s terms: this test was equivalent to the STOP-bang at identifying patients who ultimately were determined to have OSA. And remember, STOP-bang tests are the gold standard. This test simply goes above and beyond thanks to the random forest. What does that mean? We believe this research can lead to enhanced predictive measurables that can serve as a trigger for patients to receive sleep tests in a similar way that the STOP-bang currently does. The only difference: this model would look at every patient in a system’s population, not just those subjectively deemed worthy of receiving a STOP-bang test. That’s the type of end-to-end care we want to bring to all patients.Dynamic Phenotype Learning (DPL) To Develop And Discover New OSA Sub-Types
While OSA impacts tens of millions of people, there are lesser common OSA sub-types that have smaller footprints, yet just as big an impact on those that suffer from them. Some subtypes, like central sleep apnea, often can’t be solved in the same way as OSA by simply treating a patient with CPAP. Instead, nuanced care and treatment pathways must be constructed to treat these folks successfully. To address this challenge, we collaborated with Kaiser Permanente Southern California (KPSC), a large integrated health system, to create a dynamic phenotypic learning model which resulted in the “Dynamic Phenotype Learning: A Novel Machine Learning Approach To Develop And Discover New OSA Sub-Types” research study. We designed the study to leverage an innovative DPL model to identify OSA subtypes and better predict clinical risk and success with therapies than current PSG testing. What did we learn? DPL methods, applied to large and comprehensive patient datasets, can identify phenotypic biomarkers with potential to take us beyond the AHI. Rather than simply trust one metric, we believe we can uncover nuanced OSA sub-types, and the treatments best suited to drive positive health outcomes within those subpopulations.Wait… What about your other Abstracts?
Well, we can’t share all the fun before the big event kicks off! Where’s the tease without a little hidden fun, after all? Stay tuned for Part 2: Sleep 2021 Abstracts Presented by EnsoData, to come after the big show!On June 11th … Dive into all the SLEEP 2021 Abstracts
Once the conference officially kicks off, we encourage you to check out the full abstracts for all 6 of our research pieces in the SLEEP 2021 conference poster hall. We’re excited for this year’s virtual event, so make sure you stop by our booth and say hello to ‘those pineapple fellas’!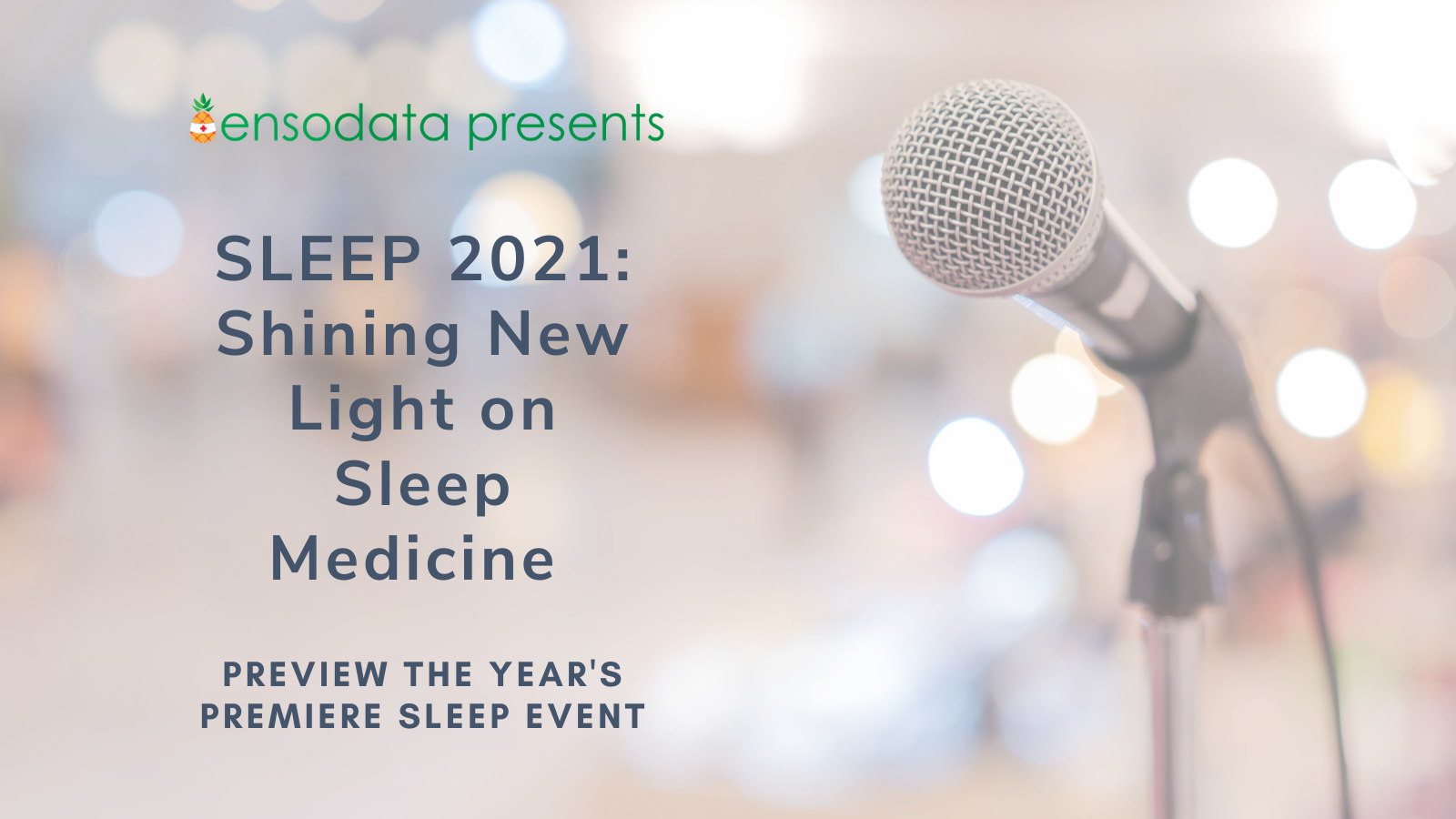